Genevera Allen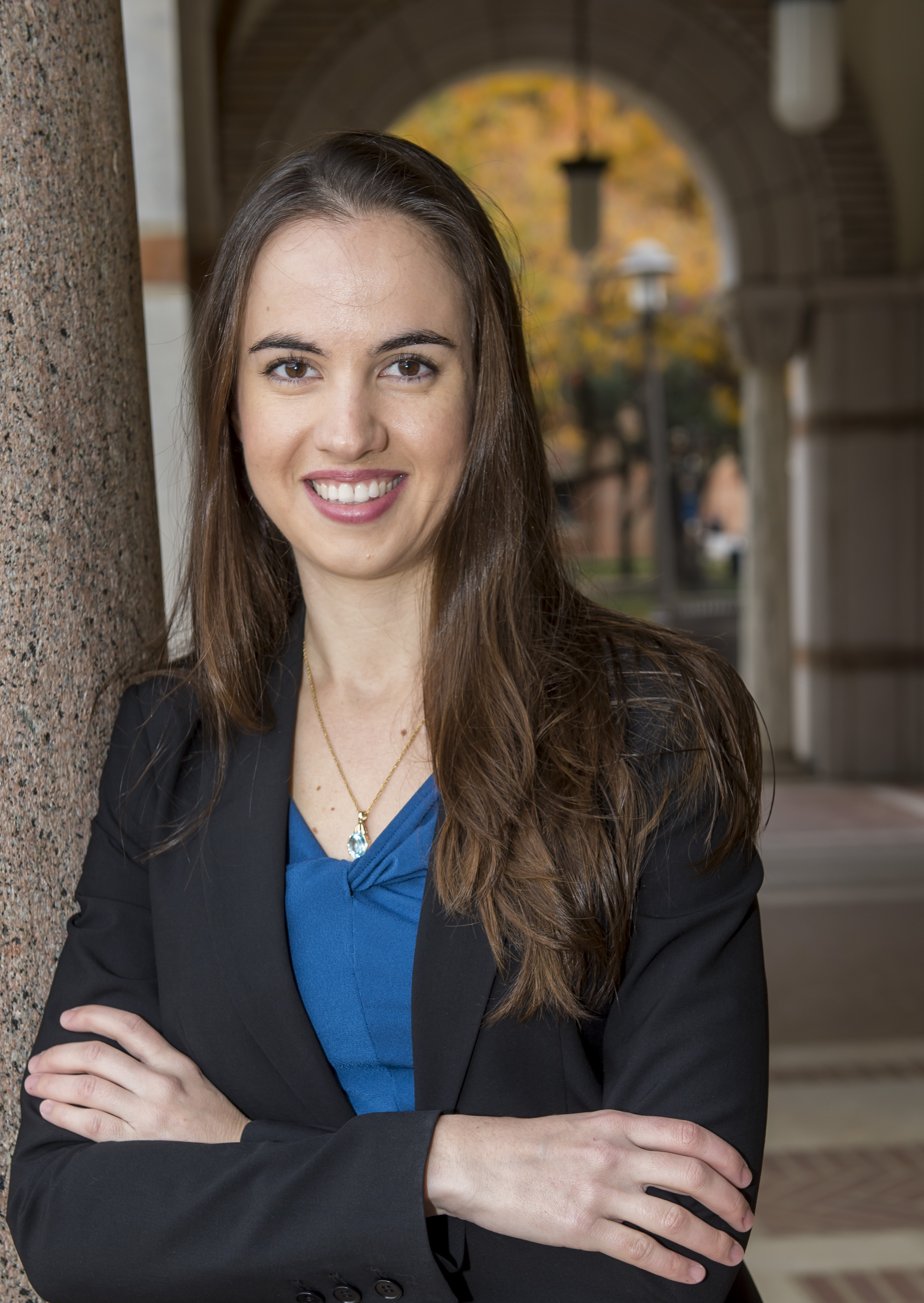
Genevera Allen is an Associate Professor of Statistics, Electrical and Computer Engineering, and Computer Science at Rice University as well as an investigator at the Neurological Research Institute at Baylor College of Medicine. Her research interests are in developing statistical tools to help scientists understand big data by using techniques from high-dimensional inference, machine learning and convex optimization. Her applied research interests include neuroimaging, neural recordings, and high-throughput genomics.
Data Integration: Data-driven discovery from diverse data sources
Genevera Allen1, 2
1Department of Electrical and Computer Engineering and Departments of Statistics and Computer Science, Rice University, Houston, Texas, United States of America; 2Jan and Dan Duncan Neurological Research Institute, Baylor College of Medicine, Houston, Texas, United States of America.
Data integration, or the strategic analysis of multiple sources of data simultaneously, can often lead to discoveries that may be hidden in individual analyses of a single data source. In this talk, we present several new techniques for data integration of mixed, multi-view data where multiple sets of features, possibly each of a different domain, are measured for the same set of samples. This type of data is common in healthcare, biomedicine, national security, multi-senor recordings, multi-modal imaging, and online advertising, among others. In this talk, we specifically highlight how mixed graphical models and new feature selection techniques for mixed, multi-view data allow us to explore relationships amongst features from different domains. Next, we present new frameworks for integrated principal components analysis and integrated generalized convex clustering that leverage diverse data sources to discover joint patterns amongst the samples. We apply these techniques to integrative genomic studies in cancer and neurodegenerative diseases to make scientific discoveries that would not be possible from analysis of a single genomics data set.
Toby Johnson
Toby Johnson is a Scientific Director at GlaxoSmithKline. His work involves developing and implementing statistical genetics tools for large scale selection and validation of drug targets, and developing requisite database infrastructure for GWAS complete summary statistics for thousands of diseases and traits. His general research interests include causal inference and Mendelian randomization, inference from summary statistics, and pharmacogenomics and biomarkers.
Identifying drug targets using human genetics at scale
Toby Johnson1
1Human Genetics, GlaxoSmithKline (GSK), Stevenage, SG1 2NY, United Kingdom
Drug efficacy and safety are definitively tested in phase III trials, completed 10-15 years after “Commit to Target” (C2T) decisions. Notwithstanding high failure rates throughout the drug discovery, three-quarters of drugs with novel targets fail in phase III. This implies many therapeutic hypotheses(modulating target X to treat disease Y) proposed at C2T are wrong. Selecting target-disease pairs using human genetic studies can increase the probability of success in drug development. This is essentially a Mendelian randomization (MR) argument, and is supported by retrospective analyses of target-disease pairs that succeeded or failed. With well powered Genome-Wide Association Studies (GWAS) for thousands of human diseases, traits, and -omic phenotypes, it nonetheless remains both challenging and necessary to infer the causal genes, in a robust and high throughput manner.
I demonstrate data and compute infrastructure, and key inferential tools, developed within GSK to systematically evaluate genetic support for every target-disease pair, and applied to C2T decisions. I focus on two areas, where I show that commonly used inferential approaches have high risk of mis-inference. For MR and Phenome-Wide Association Study (PheWAS) approaches, it is important to have good tools to evaluate the “genomic context” of the instrument(s). For GWAS-expression colocalization approaches, it is important to have tools and data to evaluate the extent of “molecular pleiotropy” across genes and tissues (and ideally cell types and conditions). Underlying the novel tools and visualizations are some conceptual advances, which could be broadly applied in “post-GWAS” research to generate more robust target-disease therapeutic hypotheses.
Kari North
Dr. North is a professor of epidemiology in the UNC Department of Epidemiology and has developed a strong multidisciplinary research program evaluating the genetic epidemiology of cardiovascular disease (CVD) and associated risk factors. Dr. North leads the UNC Department of Epidemiology’s CVD Genetic Epidemiology Computational Laboratory, a collaborative assembly of faculty members, pre- and post-doctoral fellows, and staff members spanning UNC departments with collective expertise in both family- and population-based genetic epidemiological research. At the national level, Dr. North chairs the National Institutes of Heatlh CHSA study section, is an editorial board member of multiple prominent journals and serves in several elected leadership roles in The Obesity Society and in the American Heart Association Epidemiology Council. At UNC, Dr. North has been engaged with several interdisciplinary centers that foster collaborative research in genetics.
The Future of Genomic Studies Must Be Globally Representative
Kari E. North1
1Department of Epidemiology, University of North Carolina, Chapel Hill, North Carolina, United States of America.
The past decade has seen a revolution in human genetics that has empowered investigations into the biology of complex traits. Although these discoveries rely on genetic variation, association studies have overwhelmingly been performed in populations of European descent. Given the differential genetic architecture that is known to exist across populations, such bias in representation can exacerbate disparities and impact clinical guidelines and drug development. Critical variants will be missed if they are low frequency or absent in European populations. Additionally, effect sizes and their derived risk prediction scores derived in one population may not accurately extrapolate to other populations. Here we demonstrate the value of diverse, multi-ethnic participants in large studies by providing an overview of strategies to improve global representation in research and highlighting the successes of individual studies and consortia, for example PAGE, TOPMed, and CCDG, which have provided unique knowledge. Specifically, we will outline best practices for performing genetic epidemiology in multiethnic contexts, to identify effect heterogeneity and improve fine mapping, and to demonstrate how limiting investigations to single populations impairs findings in the clinical domain and for risk prediction. We argue that lack of representation of diverse populations in genetic research will result in inequitable access to precision medicine and advocate for continued, large genome-wide efforts in diverse populations to maximize genetic discovery and reduce health disparities.
Hongyu Zhao
Dr. Hongyu Zhao is the Ira V. Hiscock Professor and Chair of Biostatistics at Yale University. His research interests are the developments and applications of statistical methods in human genetics, molecular biology, drug developments, and precision medicine. Dr. Zhao is a Co-Editor of the Journal of the American Statistical Association – Theory and Methods, and was the recipient of several honors, including the Mortimer Spiegelman Award for a top statistician in health statistics by the American Public Health Association, and Pao-Lu Hsu Prize by the International Chinese Statistical Association.
Empirical Bayes methods for genetic risk prediction
Hongyu Zhao1
1Department of Biostatistics, Yale University, New Haven, Connecticutt, United States of America
Genetic risk prediction is an important problem in human genetics, and accurate prediction can facilitate disease prevention, diagnosis, and treatment. Calculating polygenic risk score (PRS) has become widely used due to its simplicity and effectiveness, where only summary statistics from genome-wide association studies are needed. Recently, several methods have been proposed to improve standard PRS by utilizing external information, such as linkage disequilibrium (LD) and functional annotations. In this presentation, we introduce empirical Bayes methods that leverage information from effect sizes, LD and other external sources to improve prediction accuracy. Compared to most existing genetic risk prediction methods, our methods do not need to tune parameters, and are computationally efficient. We demonstrate the effectiveness of our methods through their applications to a number of complex diseases in large population cohorts. This is joint work with Wei Jiang, Shuang Song, Yixuan Ye, Geyu Zhou, and others.